- February 4, 2020
- Posted by: Abhimanyu Sundar
- Category: Text Analytics
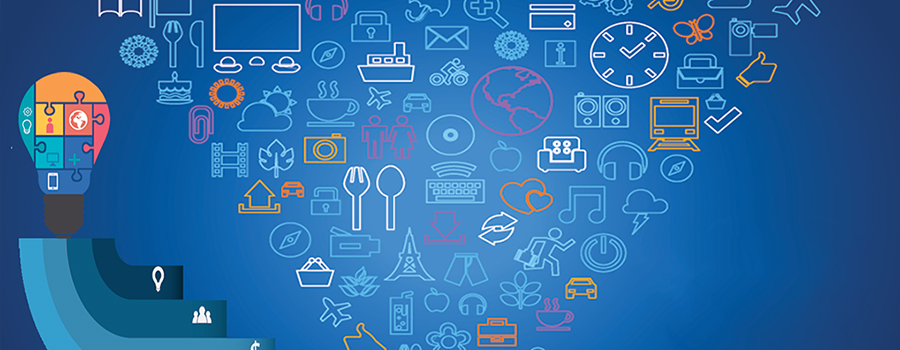
We all are in possession of loads of unstructured data. That is why 80% of the world’s data today is unstructured. The answer to all this unstructured data is text mining – which is the best way to analyze and process all this unstructured data. Most organizations today store large amounts of data on cloud platforms or data warehouses. This data grows continuously as data pours in from multiple sources. Storing, processing and analysing massive amounts of data with traditional tools becomes a challenge for organizations. In this situation, text mining techniques, text mining applications and text analytics tools come into play.
The Meaning of Text Mining
Wikipedia says – “Text mining, also referred to as text data mining, roughly equivalent to text analytics, is the process of deriving high-quality information from text.”
The definition is apt and clearly explains what text mining means – i.e. to deep dive into unstructured data in order to extract insights and patterns which are necessary to explore data sources that are textual in nature.
Text mining is a multidisciplinary field in the sense that it incorporates and integrates tools of data mining, statistics, machine learning, information retrieval and computational linguistics. The main area that text mining deals with is texts in natural language either stored in unstructured or semi-structured formats.
There are Five Key Steps in Text Mining:
- Collecting unstructured data for various sources like pdf files, blogs, e-mails, web pages, plain text and more.
- Conduct Pre-processing and cleansing of data which in turn helps detect and remove anomalies. Extraction and retention of valuable information hidden under piles of data and identification of roots of specific words is made possible with data cleansing. This can be done with a variety of text mining tools and applications.
- Conversion of relevant information into structured formats from unstructured data.
- Use the Management Information system (MIS) to analyze the patterns within the data.
- Store the insightful information in a database that is secure. This will help drive the decision-making process and also help drive trend analysis.
The Key Text Mining Techniques in Use Today
These are processes that are related to mining of text and discovering and understanding of the insights related to it. Text mining techniques generally make use of a multitude of different text mining tools and applications with the aim of perfect execution. Listed below are a bunch of famous text mining techniques that we see in use:
1. Extraction of Information
Information extraction is the most famous text mining technique in practice. This technique involves extraction of insightful information from massive chunks of textual data. The text extraction solution focuses a lot on identifying the extraction of attributes, entities, along with their relationship with unstructured or semi-structured texts. The information extracted is then stored for further analysis or use in a separate database. Precision and recall processes are used to check the efficacy and relevancy of outcomes.
2. Retrieval of Information
The retrieval of information technique is the process where extraction of relevant and associated patterns is extracted based on a particular set of phrases or words. This technique makes use of information retrieval systems that make use of various algorithms that track and monitor user behaviour and also determine related data accordingly. The biggest and most famous Information Retrieval system that all of us are aware of is Google!
3. Categorization
Text classification is a text mining technique which is a ‘supervised’ learning form where the usual language texts are set to a pre-defined bunch of topics depending on their content. Therefore, categorization or NLP (Natural Language Processing) is the process of gathering text documents for processing and analysis with the aim of uncovering the indexes or right topics for the relevant document. As a part of NLP, the co-referencing method is usually used. It is used to extract abbreviations and relevant synonyms from text data. The use cases of NLP analytics solutions have increased tremendously today and range from spam filtering to personalized commercial delivery to webpage categorization under tiered definitions.
4. Clustering
An important text mining technique is Clustering. Clustering helps identify structures that are intrinsic in nature within text information and organize them in clusters or relevant subgroups for further analysis. Forming meaningful clusters from unlabelled text data without prior information about them is one key challenge faced while performing clustering. Cluster analysis is a text analytics tool that assists with distribution of data or becomes a pre-processing stage for text mining algorithms which run on detected clusters.
5. Summarization
Multiple industries today such as academia, healthcare, law, finance and many more are being penetrated by text mining tools and techniques. This has seen a rapid rise in the rise of text mining applications. Examples of text mining applications that we see in use today are in fraud management, business intelligence, social media analysis, customer service and more.
This technique is where you can obtain compressed versions particular text that hold insightful information for the und-user. Text summarization solutions allows you to browse through various text sources in order to create summaries of texts which contain large amounts of information that are insightful in a concise format. This technique maintains the integrity in the meaning and intent of the original document. The summarization technique unites the many methods that use text categorization like neural networks, swarm intelligence, regression models and decision trees.